< Projects
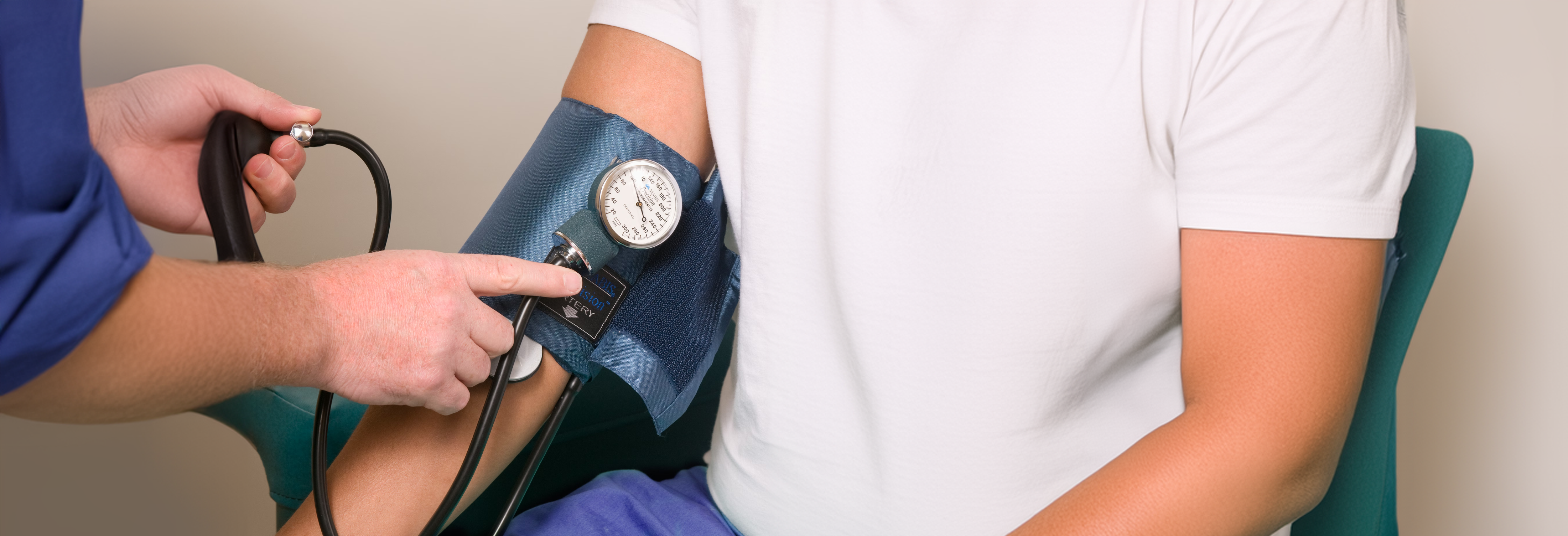
Social Complexity and Fairness in Synthetic Medical Data
As medical research increasingly relies on big data and advanced computing, privacy concerns have emerged as a significant issue, particularly when dealing with sensitive medical information. Current powerful computational methods can inadvertently compromise privacy by making it easier to identify individuals within a dataset. To address this, one promising solution involves using machine learning to generate synthetic data—creating a simulated dataset that retains the essential characteristics of the original data while protecting individual identities.
While this approach shows promise in theory, early findings suggest that machine learning-generated datasets often over-represent majority elements and under-represent minority groups. In the context of medical data, this could mean that synthetic datasets might disproportionately reflect ‘standard’ patients—such as white, middle-class, 35-year-old men—despite longstanding efforts in medical research to include diverse patient populations.
This project, which includes a two-year postdoctoral research position and collaboration between experts in AI and social sciences, aims to develop fairness metrics to evaluate the generation of synthetic data from a specific medical dataset. The hypothesis is that incorporating principles of intersectionality—recognizing the interconnected nature of social categorizations such as race, class, and gender—can lead to more equitable data representation.
In addition to creating these fairness metrics, the project will critically examine existing synthetic medical data to identify insights that social science can use to inform theoretical work on intersectional power dynamics in society. By doing so, the project seeks to ensure that synthetic data not only protects privacy but also fairly represents the diversity of the population it is meant to serve.
Start: 1 January 2023
End: 31 December 2024
fairness metrics, ML, synthetic data, medical data, intersectionality
Universities and institutes
Linköping University
Chalmers University of Technology
Project members
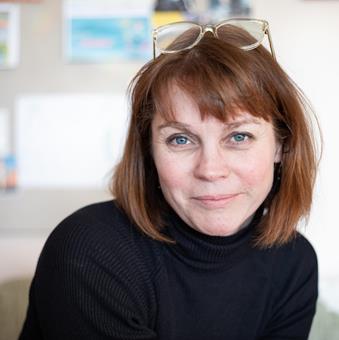
Ericka Johnson
Professor
Linköping University
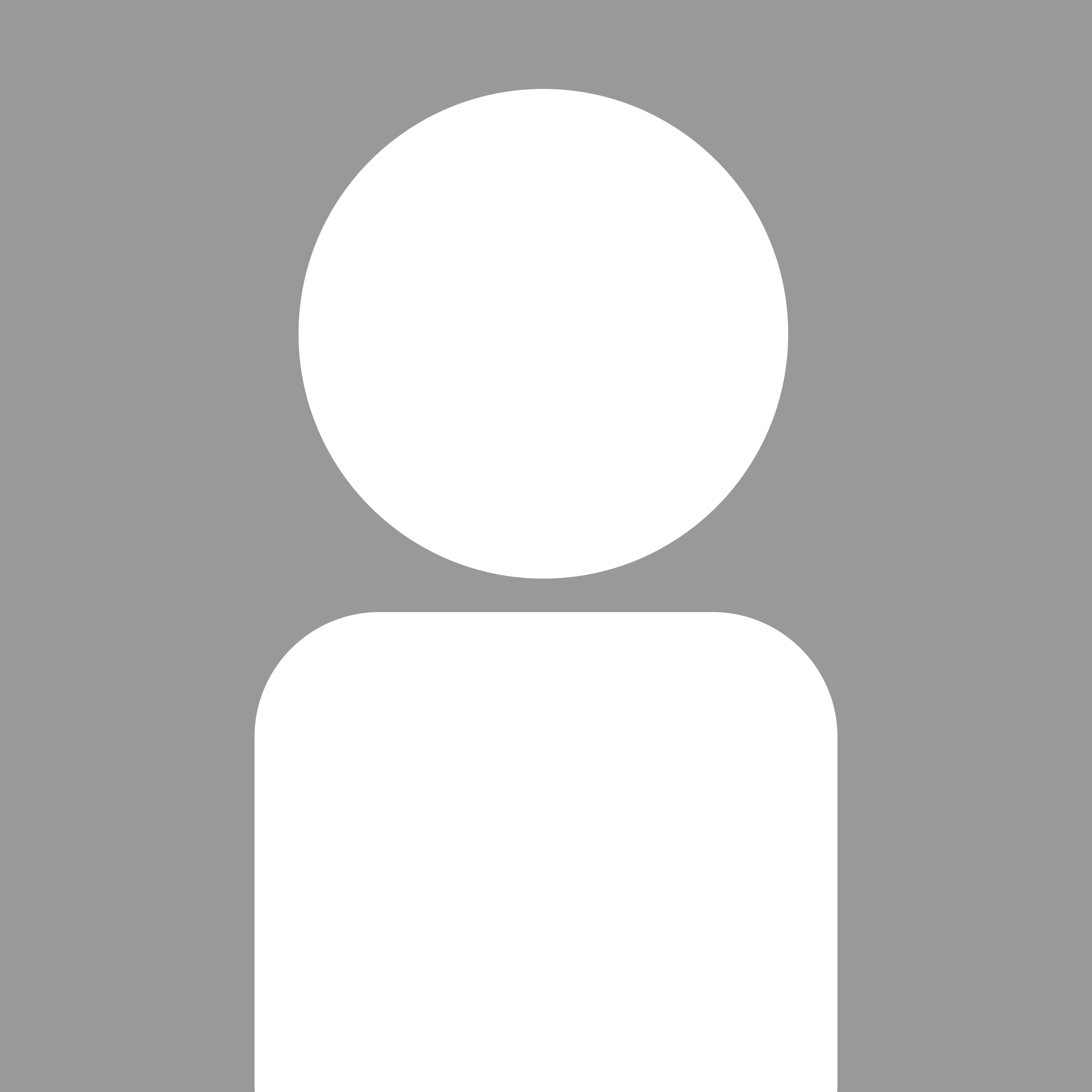
Saghi Hajisharif
Linköping University
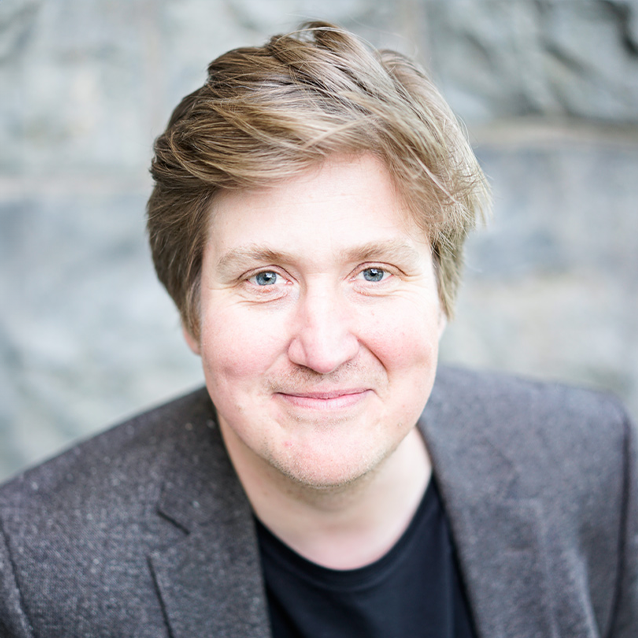
Francis Lee
Associate Professor
Chalmers University of Technology
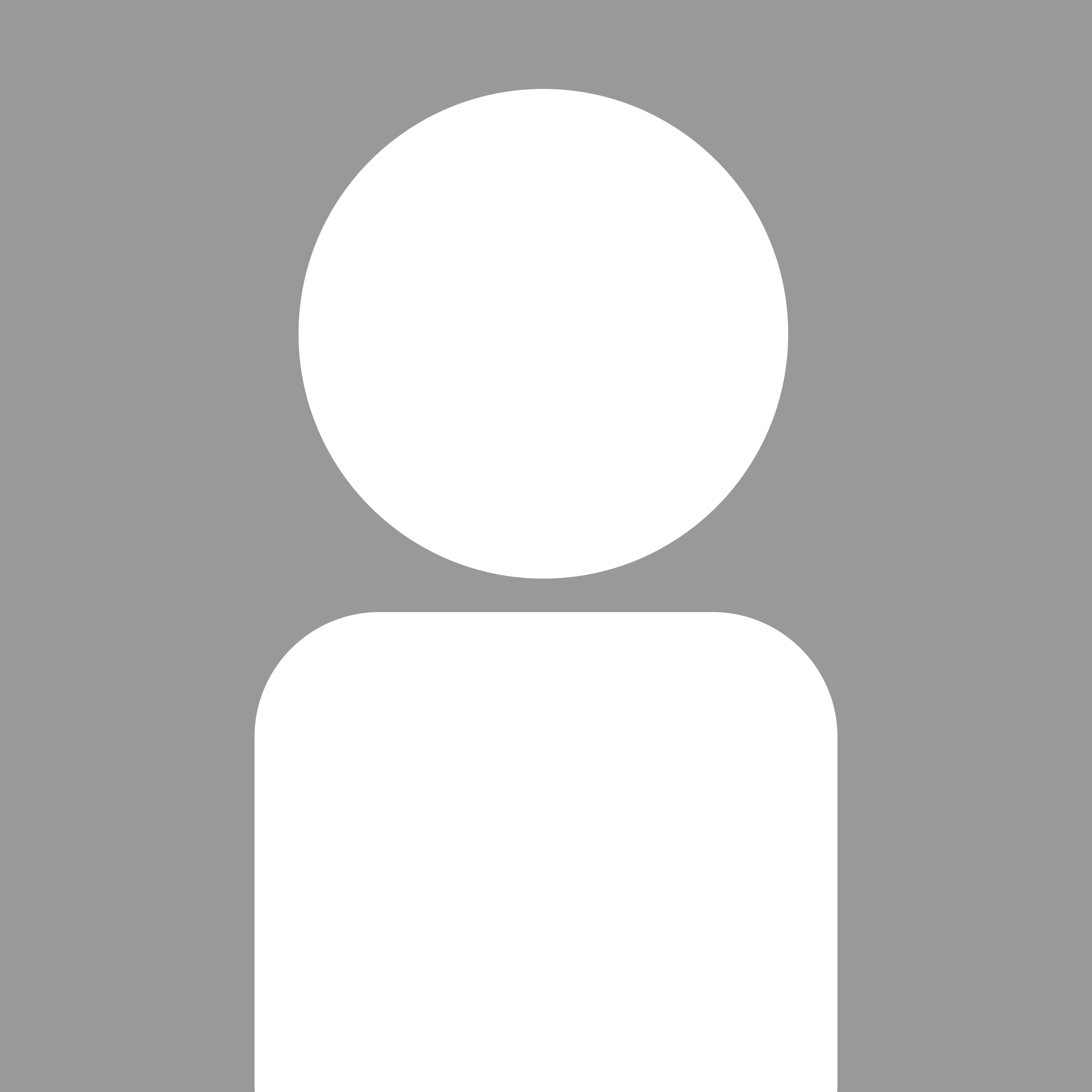
Gabriel Eilertsen
Linköping University
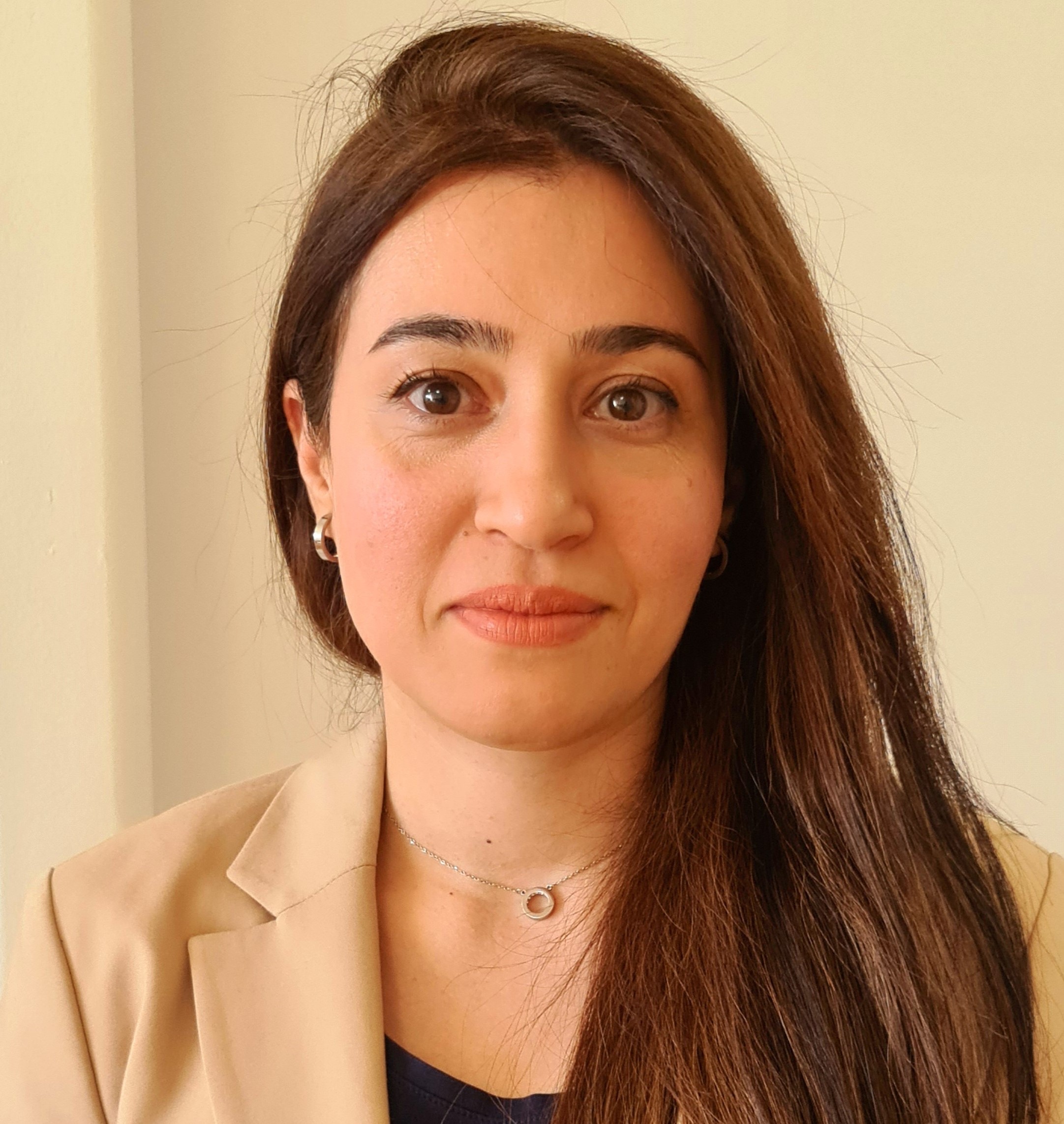
Tahereh Dehdarirad
Postdoc
Linköping University